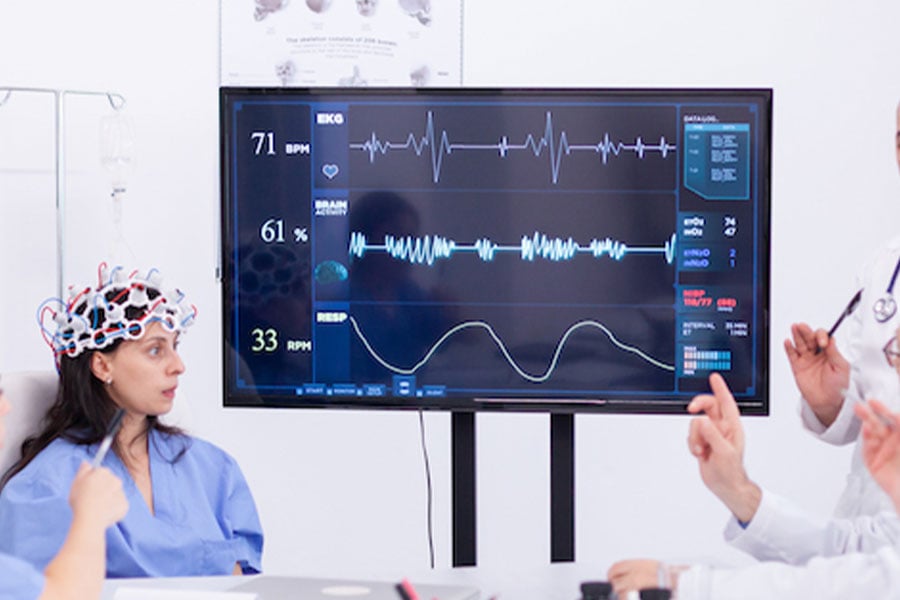
Epilepsy is a neurological disorder that is marked by sudden recurrent episodes of abnormal electrical activity in the brain, known as seizures [1]. This disease plagues more than 60 million people globally, with the same burden of disease as breast cancer in women and lung cancer in men [WHO, 2]. First line of treatment for patients with epilepsy are anti-epileptic drugs (AEDs) [3]. If AEDs are not effective in suppressing seizures, then patients may consider alternative treatments including surgical resection of the epileptogenic zone in the brain, electrical brain stimulation, or vagus nerve stimulation [4-8]. With several treatment options available, one may think that epilepsy is under control. However, this is far from true. Accurately diagnosing epilepsy and then finding an effective treatment can take years to a lifetime, during which patients and families suffer from the stigma of epilepsy, side-effects of ineffective AEDs, extensive and costly hospital stays, poor outcomes of irreversible surgical treatment, and/or less than satisfactory stimulation therapies whose efficacies are physiologically unmeasurable.
NCSL aims to establish novel EEG biomarkers and computational tools that will enable rapid and accurate diagnosis of epilepsy followed by a rapid path to an effective treatment. Such a program entails major advances in conceptual knowledge of how epileptic cortical networks behave and change during stimulation treatment addressing the following challenges:
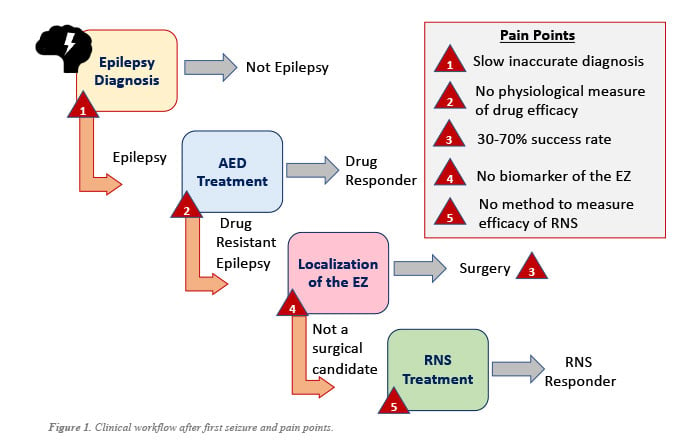
(Challenge 1) DIAGNOSING EPILEPSY MORE RAPIDLY: There is a great need to improve both the speed and accuracy of determining whether a patient has epilepsy from minutes of the first EEG recording captured, addressing pain point 1 shown Fig. 1. Such a breakthrough technology requires a conceptual advance in knowledge of what properties distinguish an epileptic brain from a nonepileptic brain and how to detect these properties from noninvasive scalp EEG data.
There are many challenges with diagnosing and treating epilepsy that unfolds as one considers the clinical workflow beginning with a patient’s first seizure (see Fig. 1). After the first seizure or seizure-like episode, patients undergo comprehensive clinical history reports, neurological examinations, and scalp electroencephalography (EEG) to obtain an epilepsy diagnosis [4,9,11]. However, the sensitivity of a routine 30-minute scalp EEG for detecting abnormalities indicating epilepsy, such as interictal (between seizure) epileptiform discharges (IEDs), varies from 29-55% [10,11], resulting in delayed diagnosis (false negative). Untreated epilepsy comes with increased risks of seizure recurrence and status epilepticus [14,15]. To reach a sensitivity of 92%, 4 routine EEGs are typically needed, which results in high economic and logistical costs, and extensive delayed treatment [15].
Misdiagnosis (false positive) often occurs in patients with psychogenic non-epileptic seizures (PNES) and syncope. In fact, as many as 25-35% of patients undergoing 24-hour video EEG in epilepsy monitoring units have PNES and are occupying costly beds with no chance of proper treatment [16,17]. Misdiagnosis also carries with it all the handicaps of a diagnosis of epilepsy in terms of stigma and social marginalization, lifestyle limitation, employment and driving restrictions, and adverse reactions to AEDs [16,17,18].
(Challenge 2) RAPIDLY MEASURING EFFICACY OF DRUGS: The process to be diagnosed as drug-resistant is trial-and-error and takes years, requiring patients to deal with a multitude of adverse side effects with no real quantifiable measures of efficacy except for patient reporting of seizure frequency. There is a great need to identify a reliable quantitative measure of AED efficacy from readily available scalp EEG data to assess efficacy more quickly, ideally within hours, addressing pain point 2 in Fig.1.
Once diagnosed with epilepsy, clinicians begin treatment with seizure preventing medications [19]. An ideal AED treatment is one that is well tolerated, fully controls the patient’s seizures, is safe, and is easy for patients to take and doctors to prescribe [20]. At present there are over 28 AEDs available in the United States, and although these provide more choices, they also increase the difficulty for clinicians and patients to quickly identify the optimal AED treatment [21]. Most patients start with a single drug where treatment dosages are gradually increased until an optimal clinical response is achieved [19]. Initial treatment is successful in ~50% of patients, and of those requiring administration of a second drug, 36.7% are successful [22]. After two failed attempts, a third drug is tried, and if it still fails to control the seizures, then clinicians will consider a combination of drugs [23,24]. Though there are no clear guidelines, presently if a patient fails all three monotherapies and a combinational treatment, the patient is then diagnosed with drug resistant epilepsy [25]. In the end, 30% will find out after several years of treatment attempts that they have drug-resistant epilepsy [26,27].
(Challenge 3) DISCOVERING ROBUST EEG MARKERS OF THE EZ TO QUICKLY LOCALIZE AND SUCCESSFULLY TREAT: There is a great need to discover and clinically validate EEG markers of the EZ, preferably from minutes or hours of intracranial EEG data and without the need to observe seizures. Such a biomarker requires a conceptual advance in knowledge of what properties distinguish the EZ from the rest of the brain and whether these properties can be captured precisely from iEEG recordings, addressing pain point 4.
Surgery is a hopeful alternative for seizure freedom in drug resistant patients, but can only be achieved through complete resection or disconnection of the epileptogenic zone (EZ), the brain region(s) where seizures originate [28]. If the EZ is focal, then surgery is an option. Unfortunately, surgical success rates vary between 30%-70% because no clinically validated biological markers of the EZ exist (pain point 3) [28-32]. Localizing the EZ has thus become a costly and time-consuming process during which a team of clinicians obtain imaging data (e.g., typically MRI, PET) and scalp EEG recordings in the Epilepsy Monitoring Unit (EMU). In many cases, lesions are identified from MRI scans (EMU Phase 1) and patients can go straight to surgery, however, in roughly half the patient pool, no lesions are visible and invasive monitoring is required, which involves days-to-weeks of capturing intracranially EEG (iEEG) as part of EMU Phase 2 [33,34]. Clinicians wait for seizures to occur and then visually inspect iEEG data, looking for abnormal activity on individual channels occurring immediately before seizures and abnormal spikes that occur between seizures.
(Challenge 4) MEASURING EFFICACY OF RNS: The the EEG recordings obtained from the RNS system should be used to continuously track the condition of the brain (baseline, pre-seizure, seizure) and to measure the effect of the stimulation when applied. Does the brain return to baseline or remain in a risky pre-seizure state? Can we adapt the stimulation pattern to steer the brain to baseline once we see that it is moving into a pre-ictal state, avoiding seizures altogether? Answers to these questions address pain point 5 in Fig. 1.
Even when the EZ is localizable, clinicians may find that surgery is not an option or cannot completely treat the patient (e.g., when the EZ is in eloquent cortex, such as visual or motor cortex). For these patients, electrical stimulation is an alternative. The Responsive NeuroStimulation (RNS) system was FDA approved in 2015 and monitors intracranial EEG in the vicinity of the EZ continuously. When it detects a possible seizure, the system stimulates the area with a burst of current pulses to stop the seizure. RNS has proven to have great potential, reducing seizures in about 50% of patients [5,6]. But RNS can do better if placed correctly, which requires accurate localization of the EZ (Challenge 3), and if the stimulation pattern is effective. Currently, there is no way to predict responsiveness to RNS treatment prior to implantation of the system, and there is no way to measure efficacy of RNS treatment after implantation except with patient logs of seizure frequency.
References
- Reséndiz-Aparicio JC, Pérez-García JC, Olivas-Peña E, et al. Clinical guideline: definition and classification of epilepsy. Rev Mex Neuroci. 2019;20(2):63-68.
- Kale R. “Bringing epilepsy out of the shadows: Wide treatment gap needs to be reduced,” BMJ 1997; 315 :2 doi:10.1136/bmj.315.7099.2
- Perucca, E. Beghi, O. Dulac, S. Shorvon, and T. Tomson, “Assessing risk to benefit ratio in antiepileptic drug therapy.” Epilepsy Research, vol. 41, no. 2, pp. 107-139, 2000, doi: 10.1016/s0920-1211(00)00124-8.
- Kwan P, Schachter S, Brodie MJ. Drug-Resistant Epilepsy. N Eng J Med (2011): 365:919-926
- Lee B, Zubair MN, Marquez YD, Lee DM, Kalayjian LA, Heck CN, Liu CY. A single-center experience with the NeuroPace RNS system: a review of techniques and potential problems. World neurosurgery. 2015 Sep 1;84(3):719-26.
- Skarpaas TL, Jarosiewicz B, Morrell MJ. Brain-responsive neurostimulation for epilepsy (RNS® System). Epilepsy research. 2019 Jul 1;153:68-70.
- Ben-Menachem E. Vagus-nerve stimulation for the treatment of epilepsy. The Lancet Neurology. 2002 Dec 1;1(8):477-82.
- Benbadis, S. R., S. Beniczky, E. Bertram, S. MacIver, and S. L. Moshe. 2020. ‘The role of EEG in patients with suspected epilepsy’, Epileptic Disord, 22: 143-55.
- Bernheimer, R. , S. O’Neal, and M. Kellogg. 2018. ‘Analysis of EEG Use Utilizing the National Inpatient Sample (P3.263)’, Neurology, 90 (15 Supplement)
- Fountain, N. B., P. C. Van Ness, R. Swain-Eng, S. Tonn, C. T. Bever, Jr., Panel American Academy of Neurology Epilepsy Measure Development, and Process the American Medical Association-Convened Physician Consortium for Performance Improvement Independent Measure Development. 2011. ‘Quality improvement in neurology: AAN epilepsy quality measures: Report of the Quality Measurement and Reporting Subcommittee of the American Academy of Neurology’, Neurology, 76: 94-9.
- Gavvala, J. R., and S. U. Schuele. 2016. ‘New-Onset Seizure in Adults and Adolescents: A Review’, JAMA, 316: 2657-68.
- Pillai, J., and M. R. Sperling. 2006. ‘Interictal EEG and the diagnosis of epilepsy’, Epilepsia, 47 Suppl 1: 14-22.
- Fisher, RS. Imitators of Epilepsy. New York: Demos; 1994.
- Oto, M. ‘The misdiagnosis of epilepsy: Appraising risks and managing uncertainty.’ Seizure Volume 44, January 2017, Pages 143-146
- Salinsky, M., R. Kanter, and R. M. Dasheiff. 1987. Effectiveness of multiple EEGs in supporting the diagnosis of epilepsy: an operational curve, Epilepsia, 28: 331-4.
- Chadwick, D. Smith. The misdiagnosis of epilepsy: the rate of misdiagnosis and wide treatment choices are arguments for specialist care of epilepsy.’ (Editorials). Br Med J, 324 (March (7336)) (2002), pp. 495-497
- Xu, D. Nguyen, A. Mohamed, C.Carcel, Q. Li, M.A. Kutlubaev, et al. Frequency of a false positive diagnosis of epilepsy: a systematic review of observational studies. Seizure (October) (2016), pp. 167-174
- A. Chowdhury, L. Nashef, R.D.Elwes Misdiagnosis in epilepsy: a review and recognition of diagnostic uncertainty. Eur J Neurol, 15 (October (10))(2008), pp. 1034-1042
- Goldenberg MM. Overview of drugs used for epilepsy and seizures: etiology, diagnosis, and treatment. P T. 2010 Jul;35(7):392-415. PMID: 20689626; PMCID: PMC2912003.
- Nunes V D, Sawyer L, Neilson J, Sarri G, Cross J H. Diagnosis and management of the epilepsies in adults and children: summary of updated NICE guidance BMJ 2012; 344: e281 doi:10.1136/bmj.e281
- Schmidt and S. C. Schachter, “Drug treatment of epilepsy in adults.” BMJ, vol. 348, 2014, doi: 10.1136/bmj.g254.
- Park KM, Kim SE, Lee BI. Antiepileptic Drug Therapy in Patients with Drug-Resistant Epilepsy. J Epilepsy Res. 2019 Jun 30;9(1):14-26. doi: 10.14581/jer.19002. PMID: 31482053; PMCID: PMC6706642.
- Hanaya R, Arita K. The New Antiepileptic Drugs: Their Neuropharmacology and Clinical Neurol Med Chir (Tokyo). 2016 May 15;56(5):205-20. doi: 10.2176/nmc.ra.2015-0344. Epub 2016 Mar 2. PMID: 26935782; PMCID: PMC4870175.
- Mervyn J Eadie (2012) Shortcomings in the current treatment of epilepsy, Expert Review of Neurotherapeutics, 12:12, 1419-1427, DOI: 10.1586/ern.12.129
- Glauser T, Ben-Menachem E, Bourgeois B, Cnaan A, Guerreiro C, Kälviäinen R, Mattson R, French JA, Perucca E, Tomson T; ILAE Subcommission on AED Guidelines. Updated ILAE evidence review of antiepileptic drug efficacy and effectiveness as initial monotherapy for epileptic seizures and syndromes. Epilepsia. 2013 Mar;54(3):551-63. doi: 10.1111/epi.12074. Epub 2013 Jan 25. PMID: 23350722.
- Kwan P, Brodie MJ. Definition of refractory epilepsy: defining the indefinable? Lancet Neurol. 2010;9(1):27-9. Epub 2009/11/17. doi: 10.1016/S1474-4422(09)70304-7. PubMed PMID: 19914135.
- Granata et al., “Management of the patient with medically refractory epilepsy,” Expert Rev. Neurother., vol. 9, no. 12, pp. 1791–1802, 2009.