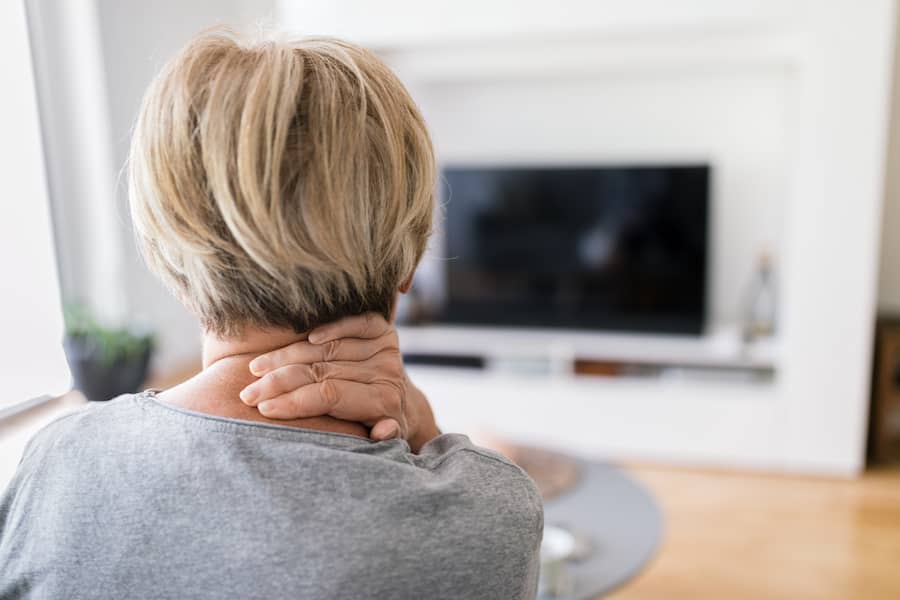
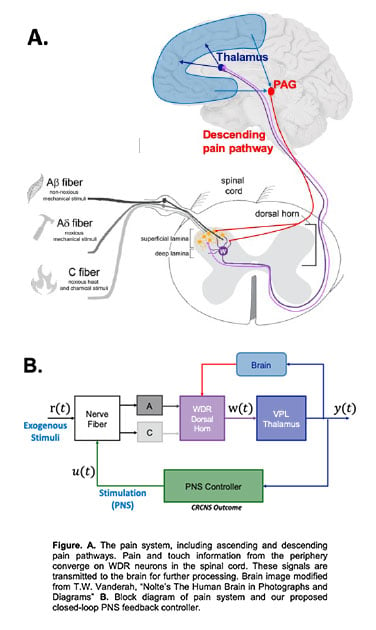
Pain is a universal experience. Acute pain is an early-warning physiological signal triggered in the nervous system, essential to detect and minimize contact with damaging or noxious stimuli. However, the nervous system responsible for the protective, nociceptive pain processing is fragile as inflammation, damage, and malfunction of the nervous system may divert its function, creating a debilitating disease known as chronic pain. Chronic pain can arise from nerve and tissue injuries. It is a significant burden on society, with an estimated prevalence of 11.2% in the U.S., and is a significant contributor to the opioid epidemic. Neuromodulation via electrical stimulation of nerve fibers, such as peripheral nerve stimulation (PNS) and spinal cord stimulation (SCS), has shown promise as an alternative pain treatment to pharmaceuticals with fewer side effects, but is still limited in efficacy for many patients. The programming of the stimulation (selective delivery of pulse width, frequency, and amplitude) is often performed by trial-and-error, and is kept constant (i.e., is open-loop) between programming sessions. Closed-loop stimulation, in contrast, adapts over time to the system’s needs by automatically adjusting the parameters in response to a measured pain signal in the body. Closed-loop approaches in engineering systems are often designed based on models that mathematically characterize how a system responds to an actuation signal. Conventional closed-loop approaches for reducing pain, however, are model-free and simply wait for measured pain activity in the spinal cord to cross a threshold before activating suppressive stimulation. This acts as a local anesthetic, suppressing pathological pain, but unfortunately, it also blocks acute pain that alerts the body to damaging stimuli.
In this research, we are addressing these limitations by building a computational framework for a novel adaptive, model-based closed-loop peripheral nerve stimulation approach for the restoration of the dysfunctional pain system back to a healthy state. This new approach will suppress long-lasting pathological (chronic) pain while allowing short-lasting protective nociceptive (acute) pain to be transmitted to the brain to be perceived. This will be accomplished by designing “model-matching” feedback control PNS strategies. For nerve-injured (neuropathic pain) animals, a closed-loop PNS controller, built using “model-matching”, will drive the response generated from exogenous mechanical stimuli (e.g., press or stroke of a paw) to mimic that of a healthy animal. In order to match responses, we first must build analytically tractable models of the response to stimulation in healthy and nerve-injured conditions by collecting data and performing system identification. We will then optimize controllers for the nerve-injured models to minimize the error between the healthy and closed-loop nerve-injured responses. These controllers will be designed and tested first in silico and then tested in vivo by continuously recording the electrophysiological response to exogenous test stimuli and adapting the therapeutic PNS applied by modulating the stimulation parameters.
The models will be estimated from novel electrophysiological recordings in naïve (healthy) and neuropathic rats in response to PNS and exogenous stimulation (mechanical and electrical) ranging from innocuous to noxious. Specifically, we will record from wide-dynamic range (WDR) neurons in the dorsal horn of the spinal cord in vivo, a cell type selected for (i) its well documented deviation from its healthy baseline in pain syndromes making it a reliable physiological readout for pain, and (ii) role as the first central relay station between pain receptors in the periphery. Additionally, we will record from local field potential (LFP) which reflect activities of populations of neurons in the ventral posterolateral nucleus (VPL) of the thalamus, a gateway for pain information to enter the brain for perception. The thalamus can be accessed and recorded from using deep brain stimulation electrodes in humans, making it an ideal location for pain therapies designed for translation. VPL will thus be the feedback measurement used for our closed-loop PNS. See Figure for a schematic of the pain system and the block diagram version that incorporates the PNS controller.
Upon successful completion of these aims, we will have evidence for an effective therapy for the restoration of the healthy pain signal from chronic neuropathic pain conditions that provides a pathway for translation into humans. The long-term goal in humans is to record LFPs using a depth electrode or a deep brain stimulation (DBS) lead in the VPL thalamus which then drives the therapeutic PNS via a chronic stimulator implanted in targeted peripheral nerves.